
In the rapidly evolving world of analytics, The pairing of LLMs with a Semantic layer is becoming increasingly crucial for businesses to harness the power of their structured data. As self-serve analytics platforms continue to advance, it's essential that organizations understand how combining Large Language Models (LLMs) with Semantic layers can unlock new levels of insight and efficiency.
This blog post will explore the evolution of self-serve analytics from OLAP dashboards to tools like Tableau and Looker to cutting-edge tools like Zenlytic, all running on Snowflake. We'll also discuss some limitations faced by businesses using LLMs without proper business context and why relying solely on generated insights can be risky.
As we delve into the importance of semantic layers in business analytics, you'll learn about their definition and role within modern systems. Then, we'll examine how Zenlytic enables seamless integration between LLMs & Semantic layers for true self-serve data exploration.
Lastly, this post will touch upon the implications for data science professionals' roles as they shift towards more advanced analytical capabilities while leveraging these powerful technologies together.
The Evolution of Self-Serve Analytics
Self-serve analytics has come a long way, with advancements in technology enabling different forms and modalities.
- From OLAP dashboards to Tableau, Snowflake, and Looker.
- Each progression has allowed users more freedom to explore data without the help of technical experts.
OLAP Dashboards for Basic Self-Serve Functionality

In the early days of self-service analytics, OLAP dashboards provided basic functionality that enabled non-technical users to view data without writing custom queries.
- These tools offered limited capabilities but were an essential first step towards democratizing access to data-driven insights.
Tableau's Impact on Data Exploration Capabilities
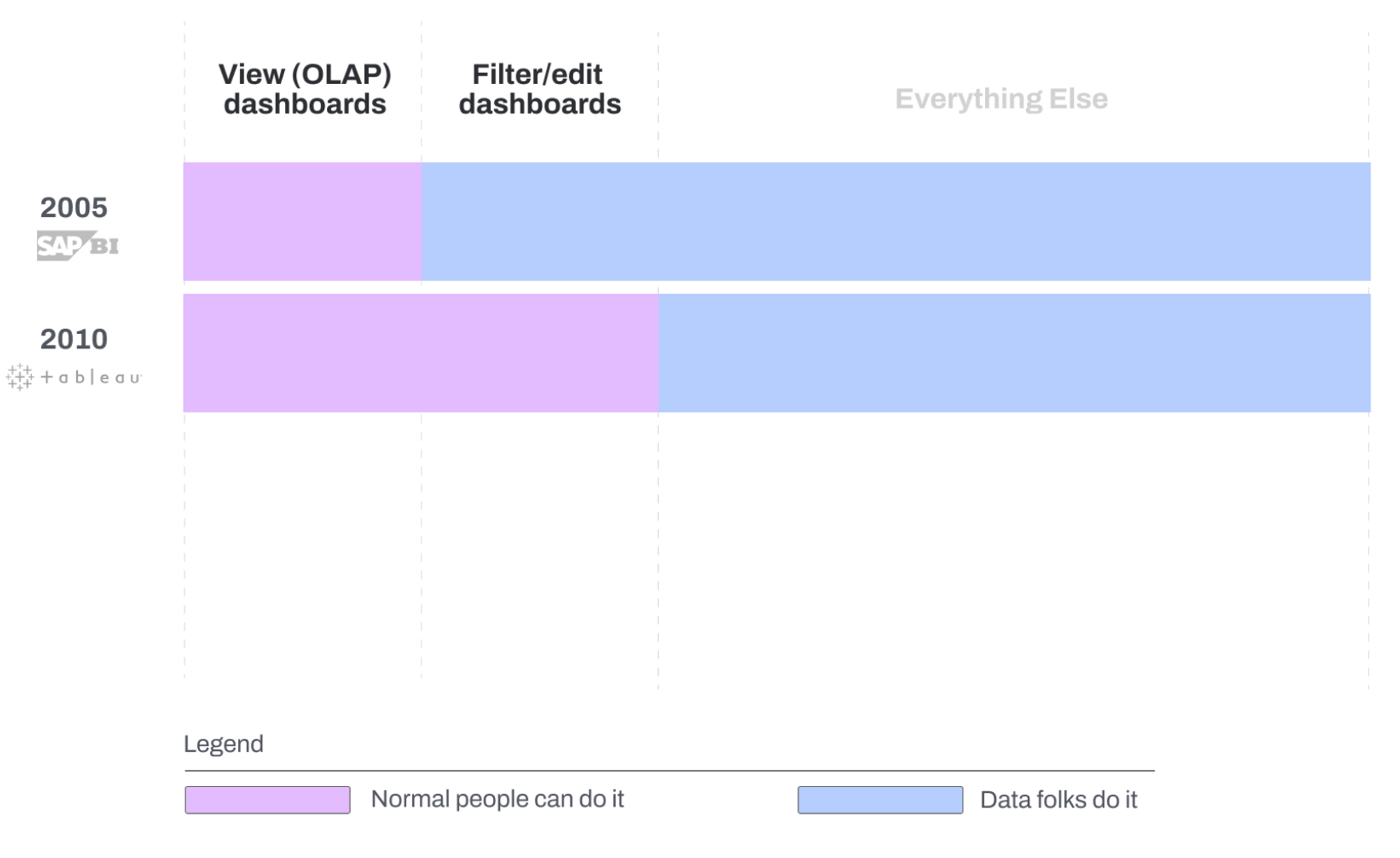
Tableau, a game-changer in the world of business intelligence, revolutionized how organizations interacted with their data.
- Providing powerful visualization features that made it easy for anyone to create interactive reports and gain insights from complex datasets.
- Finally, business users could interactively filter dashboards, something they couldn’t do with OLAP cube-based static dashboards.
Looker’s Exploration, enabled by Snowflake

The rise of cloud-based platforms like Snowflake further expanded self-service capabilities by offering scalable storage solutions combined with blazingly fast queries.
- This opened doors for products like Looker which leveraged these technologies to deliver even greater flexibility when exploring large volumes of structured or semi-structured information.
- Finally, business users could slice and dice their data to answer their follow-up questions on dashboards, which they couldn’t do in Tableau.
Limitations of Large Language Models (LLMs) Without Context
LLMs are powerful tools, and they represent the next generation of business intelligence. But without proper context, they can lead to unreliable metrics and potential legal issues.
Challenges Faced by Businesses Using LLMs Without Context
Businesses relying on Large Language Models for big data analysis might encounter several challenges due to the absence of contextual information.
- Data Inconsistency: Inaccurate or incomplete metric definitions in an LLM's output can cause inconsistencies across different departments within a company.
- Misleading Conclusions: The lack of business context may result in misleading interpretations, negatively impacting the decision-making processes.
- Potential Legal Ramifications: If incorrect numbers are reported due to insufficient contextual understanding from an LLM, companies might face regulatory penalties or even lawsuits.
Additionally, LLM-generated insights might not account for unique company-specific factors or industry trends, leading to inaccurate predictions and recommendations. These models could also generate biased results based on the training structured data used during their development.
To address these limitations and enhance the effectiveness of self-serve analytics systems powered by LLMs, incorporating a Semantic layer becomes crucial for ensuring accurate calculations every time.
The Importance of Semantic Layers in Business Analytics
A semantic layer ensures consistent metric definitions, preventing inconsistencies and improving reliability. This consistency, combined with the comprehension of the LLM enables the next generation of self service analytics.

Definition and Role of Semantic Layers in Analytics Systems
A semantic layer translates complex data structures into easily understandable terms for end-users, providing standardized definitions for metrics and dimensions.
- Data Consistency: Standardized metric definitions maintain consistency across different departments.
- Ease of Use: Users can quickly access relevant information without needing to understand complex database schemas or write SQL queries.
- Faster Decision-Making: Easy-to-understand insights enable users to make informed decisions more efficiently.
Implementing a comprehensive semantic layer alongside LLMs in self-serve analytics is crucial to unlocking deeper insights with greater accuracy.
Unleashing the Power of LLMs & Semantic Layers for Self-Serve Data Exploration
Large Language Models (LLMs) are great, but when combined with well-defined semantic layers, they become unstoppable.
With Zenlytic, companies, from startups to enterprises, can now enjoy the benefits of this powerful combination, providing accurate and consistent insights across their organization.
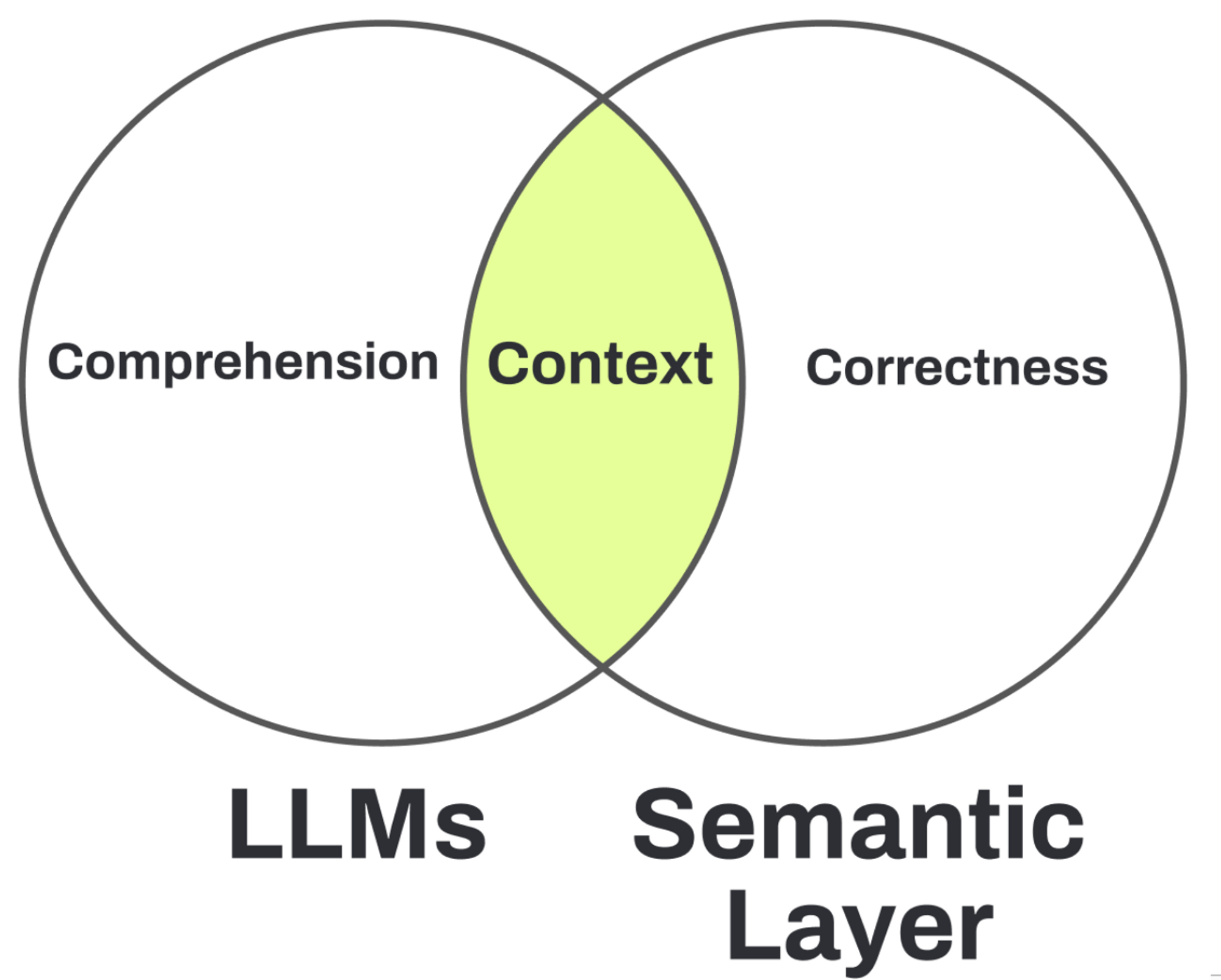
How Zenlytic Makes It Happen
Zenlytic's semantic layer ensures that all calculations are accurate every time, preventing potential legal issues due to incorrect reporting.
Non-technical users can now ask complex questions and receive reliable answers in natural language format without needing assistance from data scientists or analysts.
- Data engineers can build comprehensive semantic layer that define metrics consistently throughout the organization.
- The integrated system ensures all calculations are accurate every time, preventing potential legal issues due to incorrect reporting.
- This approach empowers non-technical users to ask complex questions and receive reliable answers in natural language format without needing assistance from data scientists or analysts.
By incorporating both LLMs and a semantic layer into your analytics system, you not only enhance self-service capabilities but also improve overall reliability and efficiency within your organization's decision-making process.
Implications for Data Science Professionals' Roles
As LLMs and semantic layers become more prevalent, data scientists will experience a shift in the nature of their work. Instead of spending time on mundane tasks like writing SQL queries, they can focus on complex statistical analyses, custom model building, and other specialized skills that require their expertise.
Shift in the Nature of Work for Data Scientists
- Data preparation: With LLMs handling basic querying tasks, data scientists can dedicate more time to cleaning and preprocessing datasets for advanced analytics.
- Analytical modeling: As self-service tools handle simpler analyses, data professionals can concentrate on developing intricate models tailored to specific business needs.
- Innovation: Freed from routine responsibilities, data scientists have an opportunity to explore new techniques and technologies that drive innovation within their organizations.
Increase Value Placed on Advanced Analytical Capabilities
The integration of LLMs with a semantic layer not only enhances self-service capabilities but also emphasizes the importance of advanced analytical skills. This development allows companies to recognize the true value provided by expert-level insights derived from complex models built by skilled professionals. Consequently, this recognition may lead to increased investment in talent acquisition and training programs aimed at nurturing these essential competencies among team members.
FAQs in Relation to LLMs & Semantic Layer
What is the Semantic Layer?
The Semantic Layer is a simplified view of complex data structures that enables non-technical users to easily explore and analyze information without needing deep technical knowledge.
What are Examples of Semantic Layers?
Examples of LLMs Semantic Layer include cube.dev, Looker's LookML, dbt’s Semantic Layer, and Power BI’s DAX.
What is the Typical Purpose of the Semantic Layer in Analytics Systems?
The primary purpose of the Semantic Layer in analytics systems is to facilitate self-service analysis by translating complex database schemas into understandable business terms.
What are the Benefits of a Semantic Layer?
- Simplified access to complex data sources
- Faster time-to-insight for end-users
- Better collaboration across teams with shared understanding
- Easier maintenance through centralized metadata management
- Improved data governance and security
Conclusion
LLMs & Semantic Layer is transforming self-serve analytics, leaving previous generations of self-service analytics in the dust.
But relying solely on LLM-generated insights can be risky without proper context, which is where the semantic layer comes in.
Zenlytic seamlessly integrates LLMs and the Semantic Layer, allowing for iterative question-and-answer experiences that increase the value of advanced analytical capabilities for data science professionals.